Loamin x Crosscut: Modernizing Malaria Prevention with Geospatial Maps
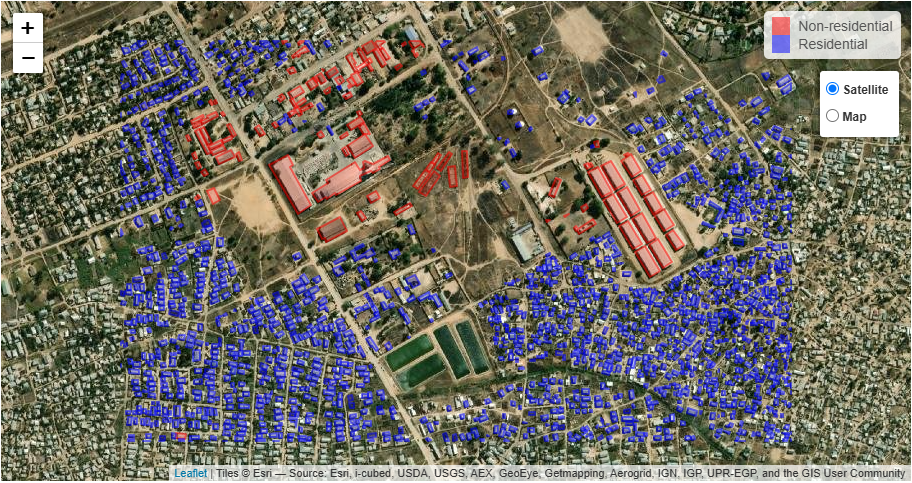
Malaria prevention teams succeed when they know exactly which structures to spray with insecticide. Yet for far too long, these dedicated community outreach teams and volunteers have relied on maps based on aged or incomplete data. These maps can miss new settlements or lead to duplicated efforts and squandered resources. As part of our work on the PMI Evolve project managed by Abt Global, we set out to tackle this challenge when Loamin and Crosscut joined forces last year.
By combining Loamin's geospatial analysis with Crosscut's microplanning tools and leveraging OpenStreetMap data, we designed simple geospatial maps that show which structures need (or do not need) spraying. I want to share how this collaboration has helped teams cover more ground, make the most of limited supplies, and protect more local communities from malaria.
The problem: Which buildings need spraying?
Indoor residual spraying (IRS) is a fundamental tool for preventing malaria. Teams equipped with backpack-mounted insecticide tanks travel from building to building, spraying walls to kill mosquitoes and prevent them from breeding. IRS is used all around the world and has significantly reduced or eliminated malaria across four continents.
But these campaigns face a fundamental challenge: identifying exactly which buildings need to be sprayed. Not every building is eligible. Homes are sprayed, but schools, factories, and other non-residential structures typically aren't. It can be costly to determine how much insecticide and other resources are needed to carry out the work if it requires going house-to-house, so there is a tradeoff that is made between cost and accuracy when planning campaigns.
A common approach relies on large paper maps that field teams review each morning to plan their daily routes. Team leaders assign different areas to different spray operators: "Team A focuses northeast, Team B covers these communities to the west." But these maps rarely reflect recent construction or settlement changes, which can create significant coverage gaps.
How we approached the solution
Our collaborative solution needed to address a specific question: Is this building a home that should be sprayed, or is it a non-residential structure that should be skipped?
Here's how we tackled it:
- Building Identification: We used OpenStreetMap to pinpoint documented structures within the target areas, creating an accurate baseline map of all known buildings. OpenStreetMap is an amazing resource for this kind of task, but it’s just the starting point, as we don’t yet know how many of those structures are actually spray-eligible residential buildings and coverage is not 100%.
- Residential Classification: This is where Loamin's expertise came in. Loamin’s machine learning algorithm analyzed structures to determine spray eligibility. The algorithm characterises each structure in terms of its shape, footprint area, proximity to roads, rivers, and neighboring buildings. It then looks for those features that distinguish residential from non-residential. For example, footprint area alone helps to identify warehouses, hospitals, and schools. Combining several building attributes pushes accuracy to roughly 85%. Once the model was trained, it generated predictions of residential/non-residential status for all structures across the country.
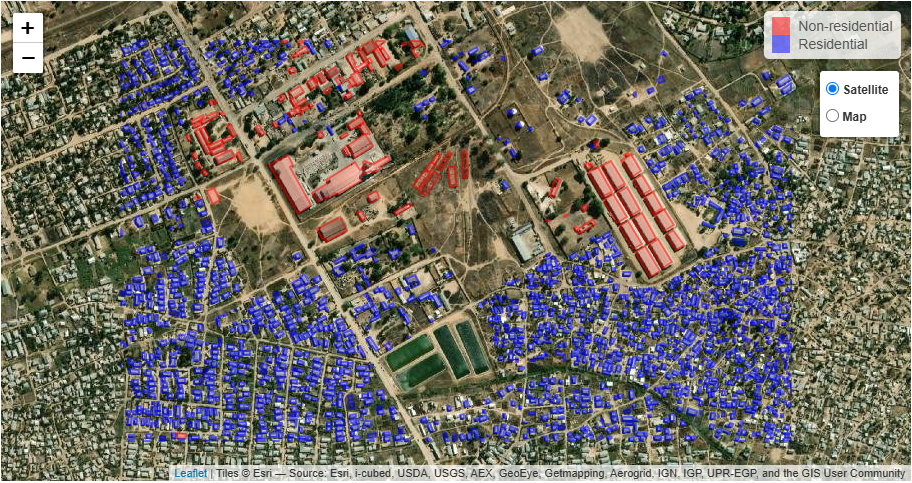
- Map Creation: The Crosscut App then generates printable PDFs that transform the geospatial analysis into easy-to-use documents that could be viewed and printed without specialized software. These maps highlighted key pieces of information such as the locations of communities with significant numbers of spray-eligible structures, health facility catchment borders, schools, and other features.
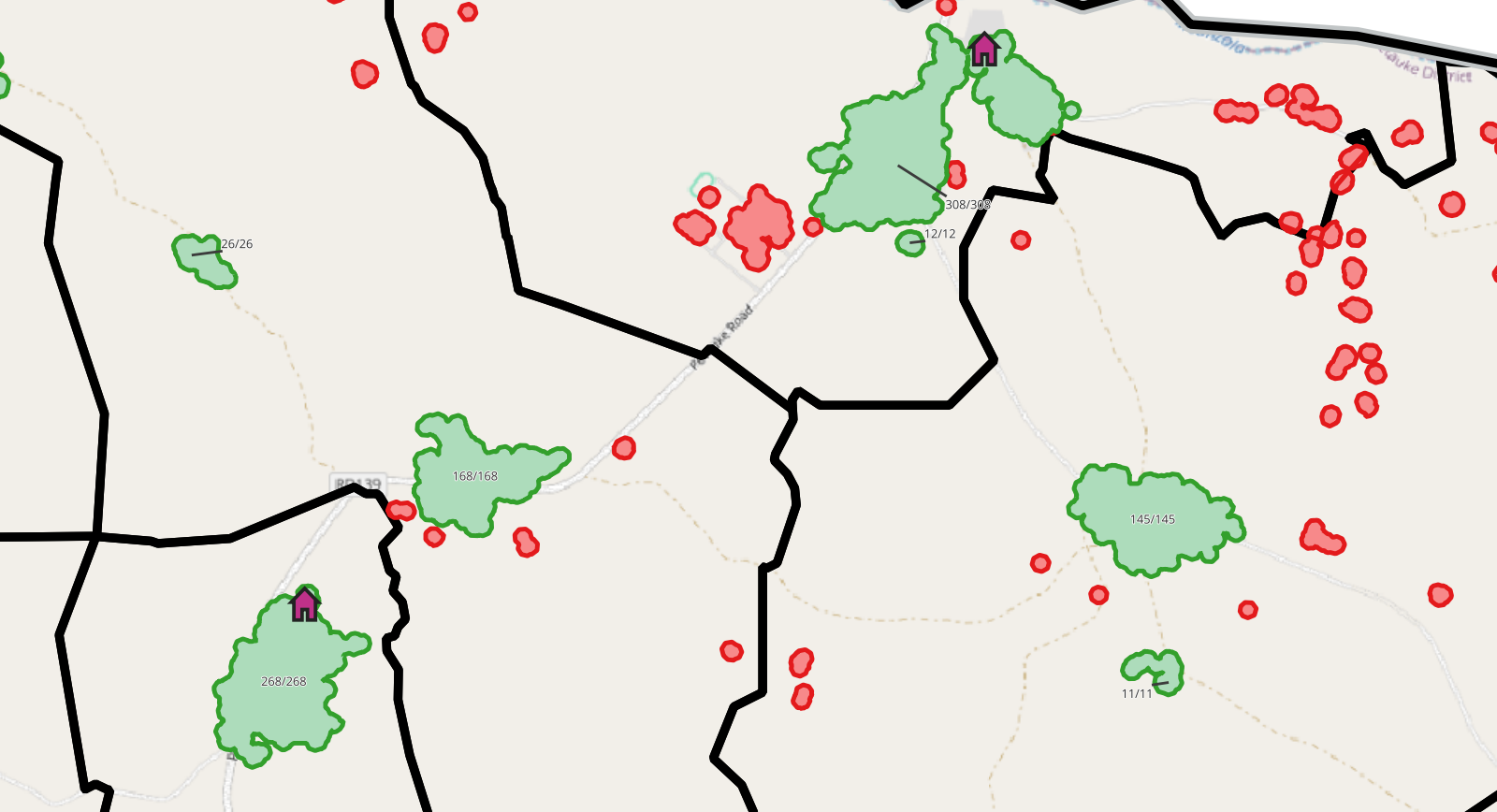
- Field-Ready Resources: The finalized maps can be printed and directly used by field teams to plan the campaign.
The impact: Better coverage, less cost
The gold standard of accuracy is to go out and confirm every single building as spray eligible. However, this is very costly and is often not possible in time for procuring supplies, hiring spray teams, and allocating resources. Loamin's machine learning approach lets us get 85+% accuracy for a fraction of the cost of a 100% building-to-building census. Having the information on easy-to-read maps help ensure all structures where people are sleeping have the coverage needed to work towards elimination of malaria.
While we haven't been able to collect comprehensive quantitative data on the impact (due to the program's timing), the client feedback was clear: having accurate, visual guidance eliminated much of the guesswork from spray operations, which should lead to larger coverage zones.
Perhaps most importantly, this approach required minimal changes to existing workflows. Teams continued their familiar planning process, just with significantly better information to guide their decisions.
What's next, key takeaways
There’s real value in combining specialized expertise to solve nagging operational challenges in public health initiatives. Some key takeaways from this partnership:
- Simple solutions (like better maps) can have outsized impacts on complex problems
- Combining geospatial analysis with microplanning provides more accurate targeting
- Physical maps remain powerful tools when designed with the right information
As climate change extends the range of mosquito-borne diseases and public health budgets tighten, purpose-built solutions like these can and will help protect vulnerable communities with increasingly limited resources.
If your organization faces similar challenges in resource allocation and geographic targeting, this approach offers a promising path forward. If you're interested in exploring how a similar solution might apply to your public health challenges, you can reach us here: James or Hugh.
Related Posts
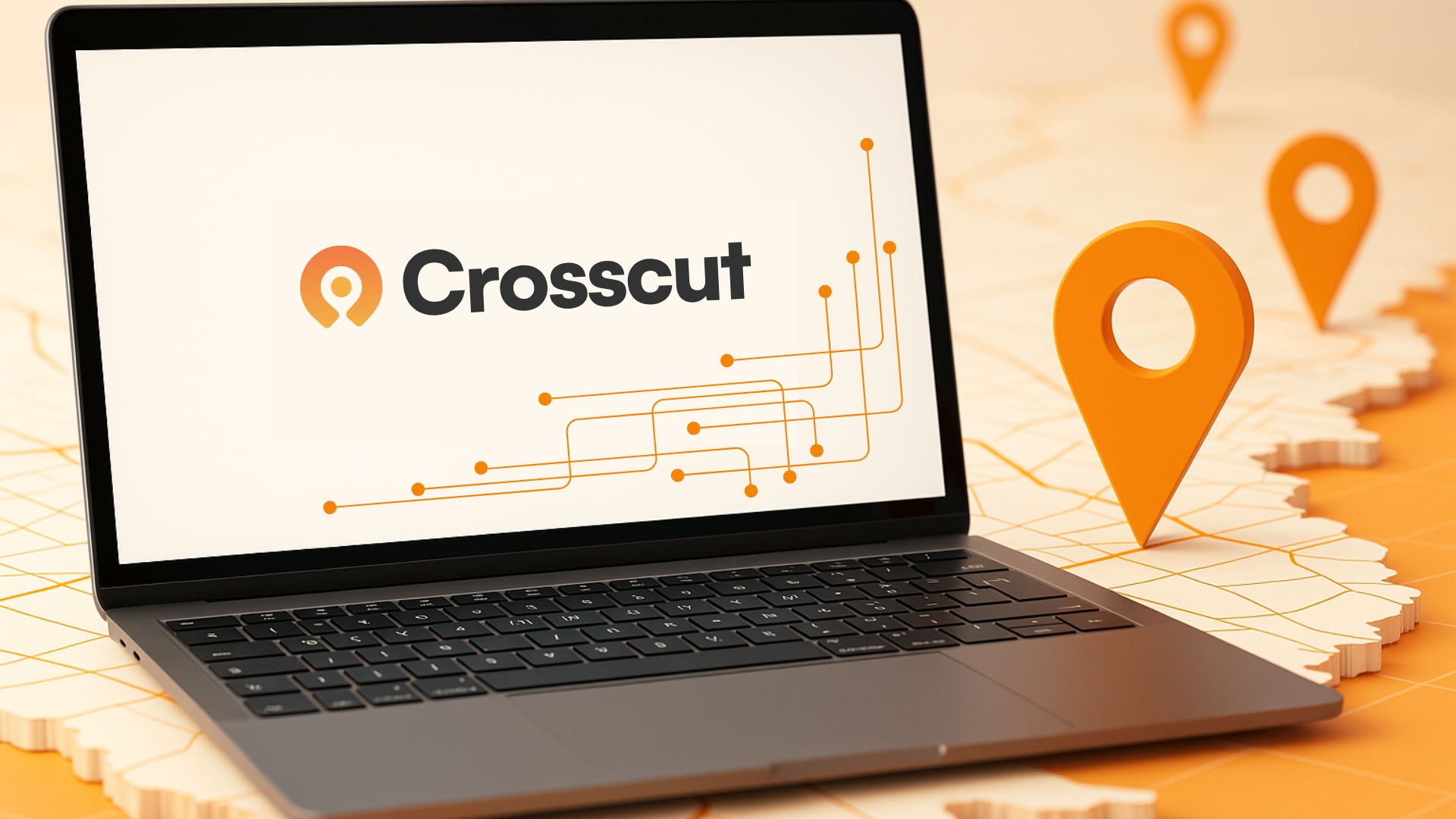
How AI Is Impacting Our Work
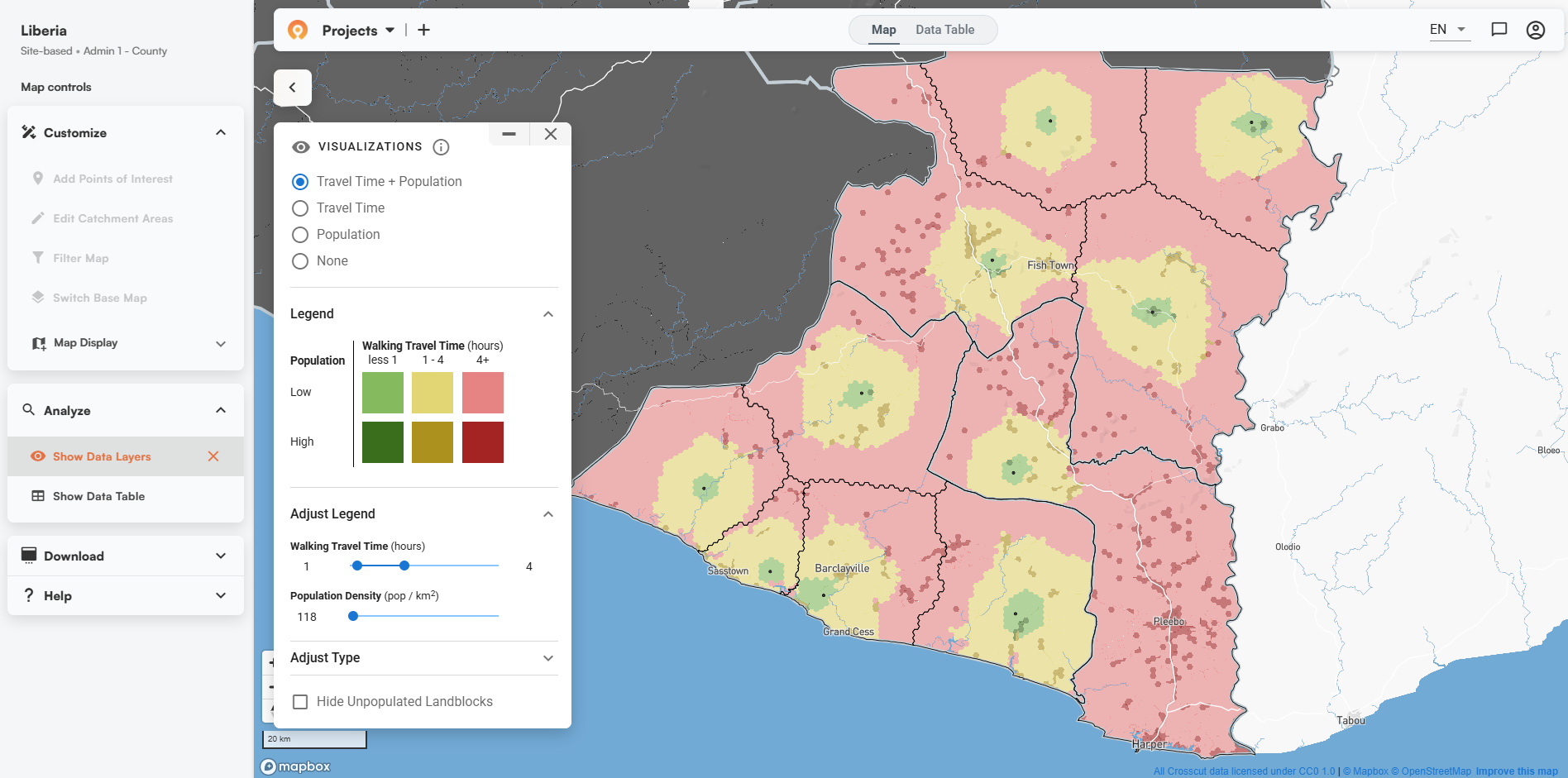
Microplanning for Malaria Control
